Artificial Intelligence application in Manufacturing 4.0 Industrial Revolution
Manufacturing Organization | 90 - 100 Employees
The investigation of intelligent problem-solving behavior and the creation of intelligent computer systems. In other words, AI describes the work processes of machines that would require intelligence if performed by humans.
AI was coined way back in the 1950’s where the assumption was that all the aspects of learning and the notable domains of intelligence can be simulated by a machine. This is part of Weak AI, and notable enhancement towards strong AI includes the ability to do self-learning which makes AI popular in the current context. Manufacturing always required pre-planning to ensure the highest quality of deliverables and economic scales. But in the real world, it does not happen which results in marginal higher cost as well as slight deterioration in Quality.
The Power of AI will help find discrepancies in each stage and data-driven insight gives the ability to make informed decisions. Illustrate some examples of common issues in manufacturing Field Service Challenges
- AI-enabled pre-build service templates allow you to quickly create new service work instructions. This reduces the service cycle.
- Full feature checklist with associated data collection will help to understand the behavior of the machine or component and will help us to derive the cost, end-of-life.
- Automated Escalation, notification, diagnostic, and post job activity help improve overall efficiency and product life and reduce wear and tear
Maintaining equipment is an essential aspect of any production, whether it is dependent upon assets or not.
Computer algorithms can be created by feeding input design goals into the generative design software and parameters such as performance or spatial requirements, materials, manufacturing methods, and cost constraints.
The algorithm examines all possibilities and generates a few optimal solutions. Various solutions can be assessed using pre-trained deep learning models, which can provide additional insights and enable confident choices to be made. Unlike humans, artificial intelligence is objective and doesn’t make unproven assumptions.
Innovative facilities generate manufacturing intelligence that impacts the entire enterprise, thanks to cutting-edge technologies such as Big Data and IoT.
Manufacturing companies can access a previously unimagined amount of sensory data in multiple forms, structures, and semantics. As a result, deep learning received significant attention as the leading innovation in computational intelligence during the transition from sensory data to manufacturing intelligence.
People can automatically learn from data, detect patterns, and make decisions with the help of deep learning techniques. It is possible to distinguish various levels of data analytics, including predictive analytics, prescriptive analytics, diagnostic analytics, and descriptive analytics.
- With predictive analytics, you can predict the possible prospects of a company’s production and equipment degradation based on statistical models.
- Diagnostic analytics tries to figure out what’s wrong.
- Descriptive analytics describes what is happening right now by analyzing operational parameters, the environment, and the conditions of the product.
Using deep learning, we can transform the manufacturing unit into a high-performance, innovative facility. This solution provides many benefits, including a decrease in operational costs, a more flexible response to customer demand, an increase in productivity, improved visibility, and a greater return on investment for the operation.
Enough historical data is required to help us understand past failures. Based on the characteristics of the system/machine and the information available, it becomes possible to analyze.
- What model should be selected?
- Is the malfunction a sudden event, or is it a gradual process?
- Is every event recorded before a failure occurs?
- How far in advance should the model be able to predict when the system will fail?
The most appropriate solution modeling strategy should be selected when all the necessary information is available. Some of the models modeled using the above scenarios will make predictions based on the question they are attempting to answer and the type of data they require.
- A regression model to predict remaining useful lifetime (RUL.
- Using classification models to predict failure within a specific timeframe.
- It is essential to have the correct data available.
- Proper framing of the issue could result in a more effective fix.
- Properly evaluate the predicted outcomes.
#arocom #artificialintelligence #machinelearning #datascience #industrialrevolution
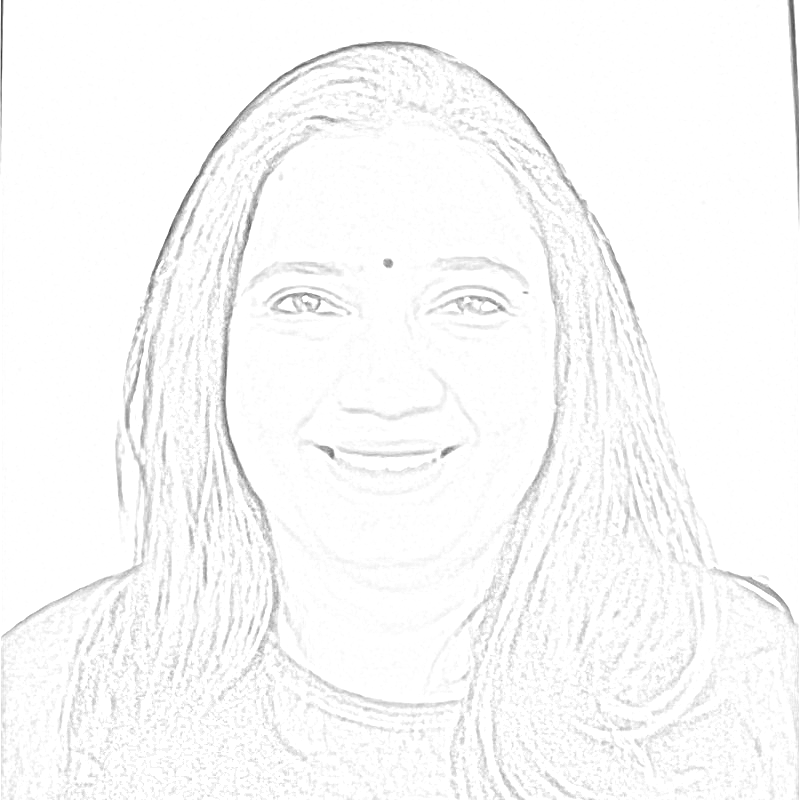
Lakshmi is a team manager at Arocom. She is enthusiastic about learning and using the latest technologies of Artificial Intelligence (AI) and Machine Learning. Her main interest is in finding areas where AI and Machine Learning can be applied to solve problems.
.